FRUTILLA
Frostvorhersage für den Obstanbau durch maschinelles Lernen und Sensorsynthese
Projektdauer
01.07.2022 - 30.06.2025
Förderprogramm
Förderkennzeichnung
031B1279
Gefördert durch
Bundesministerium für Bildung und Forschung
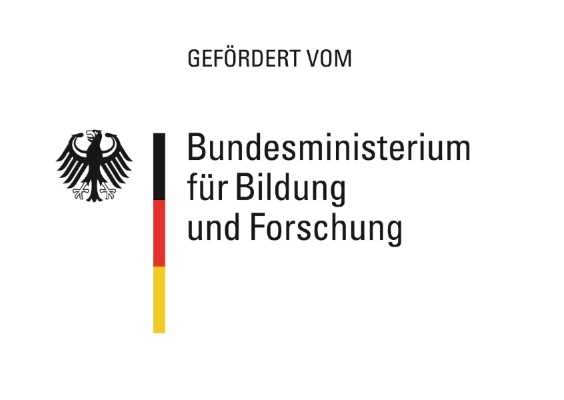
Internationales Projekt
SDG
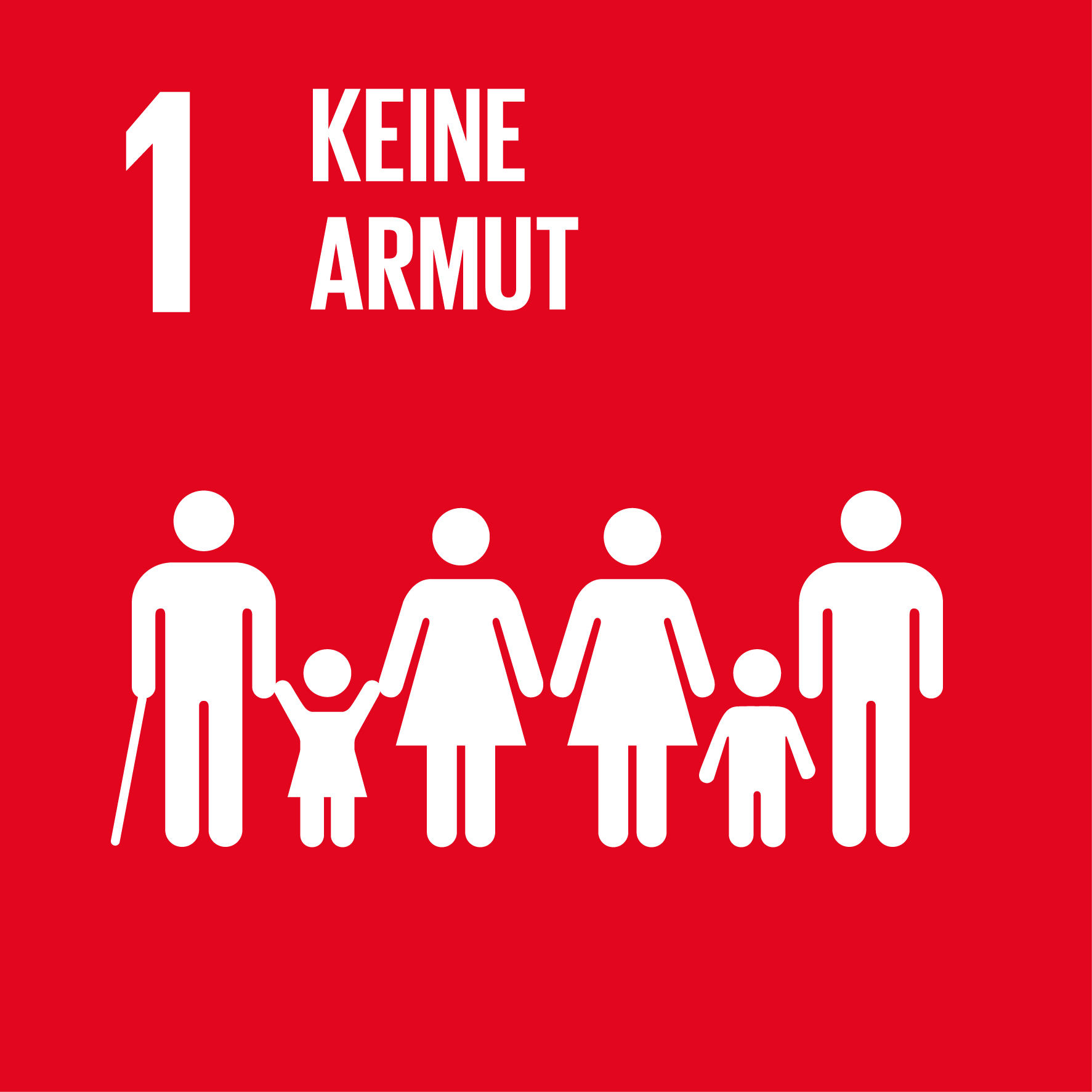
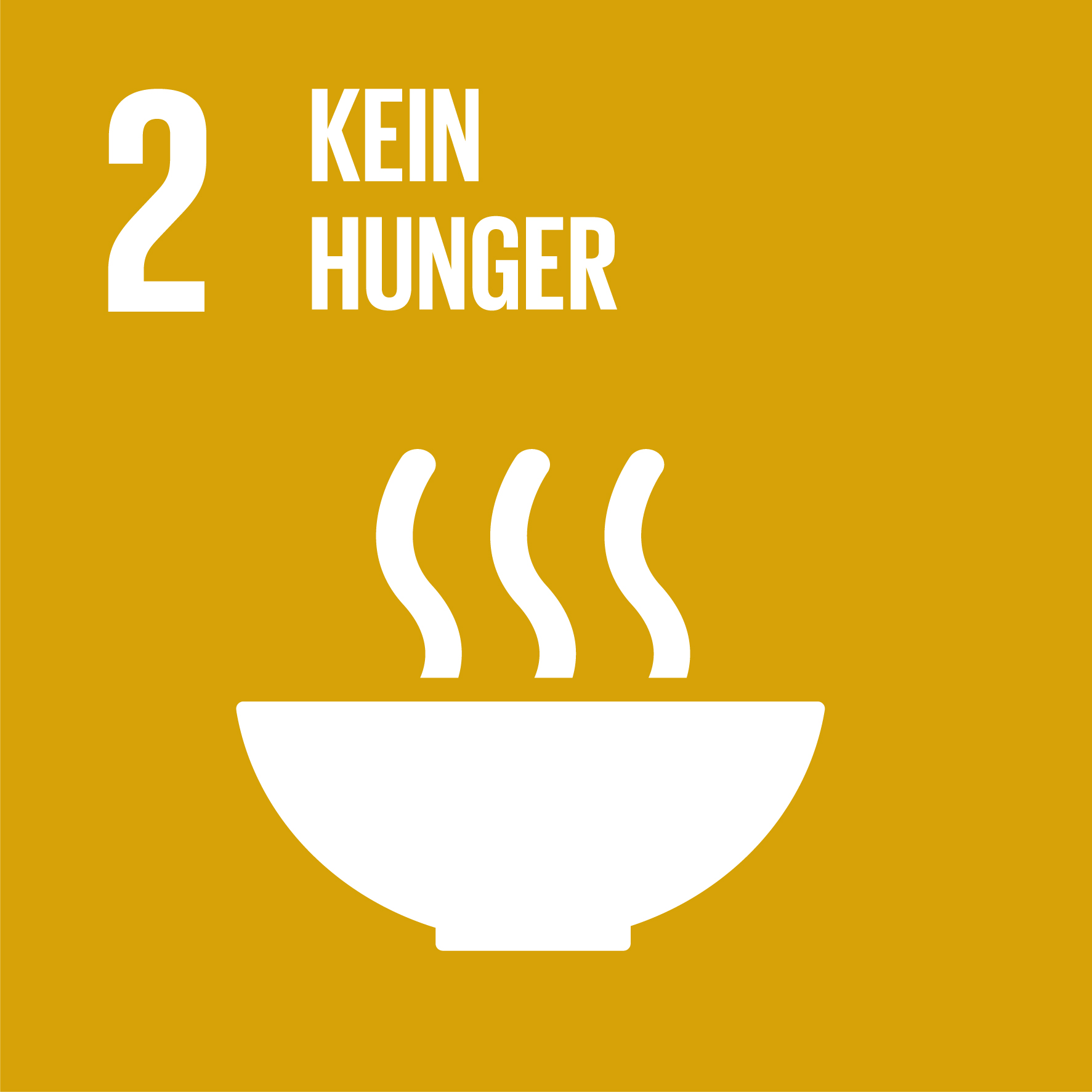
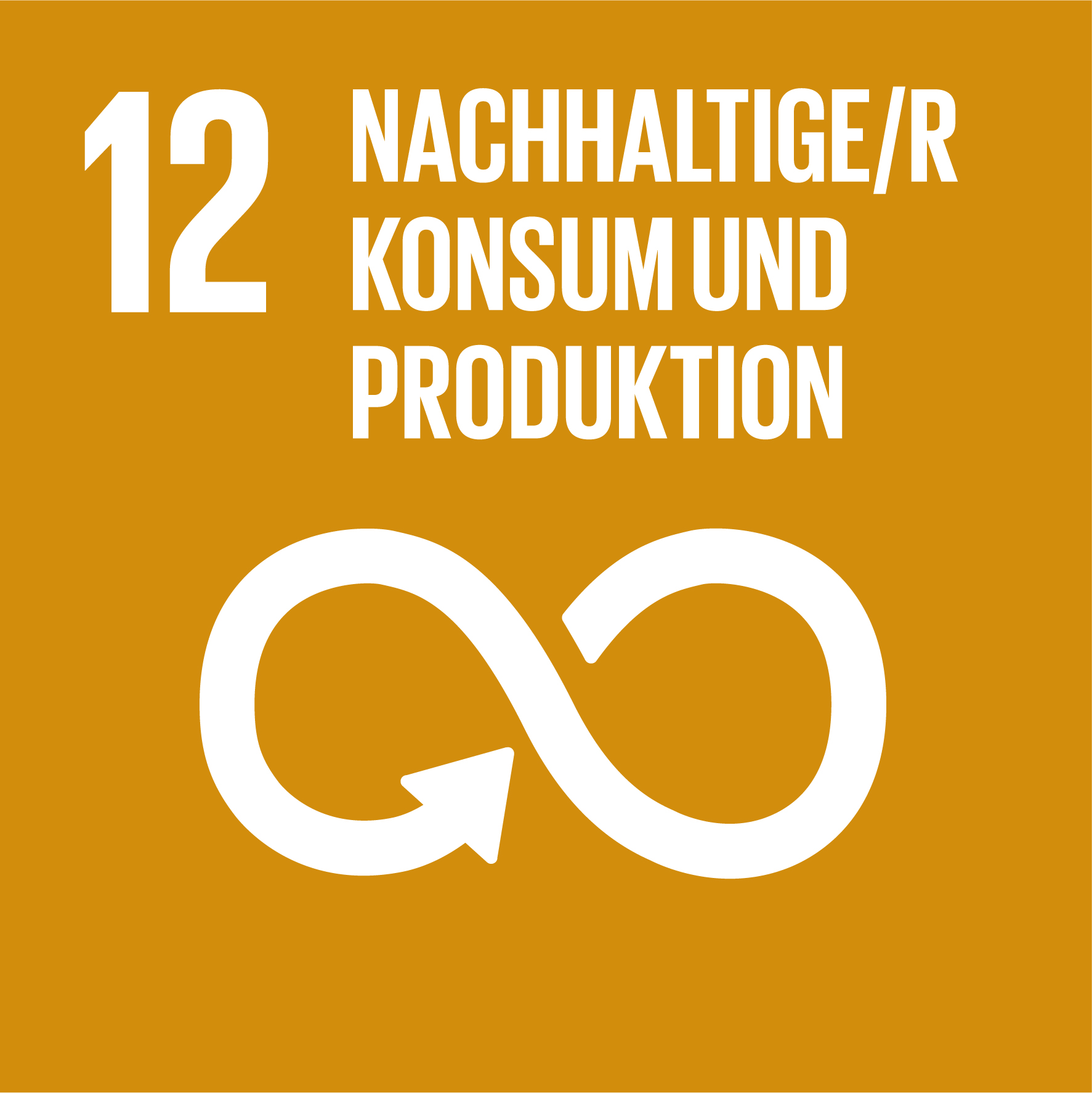
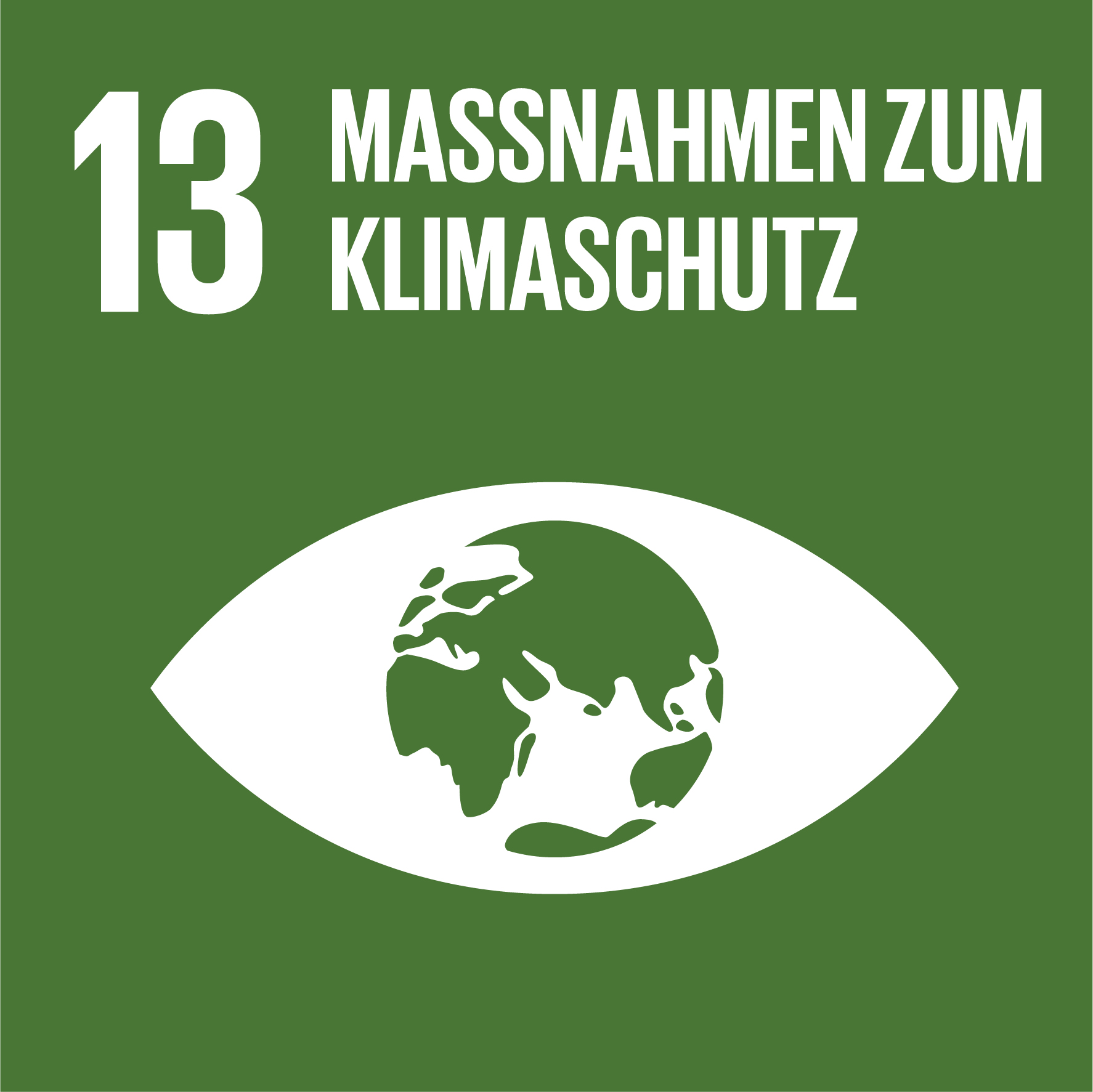
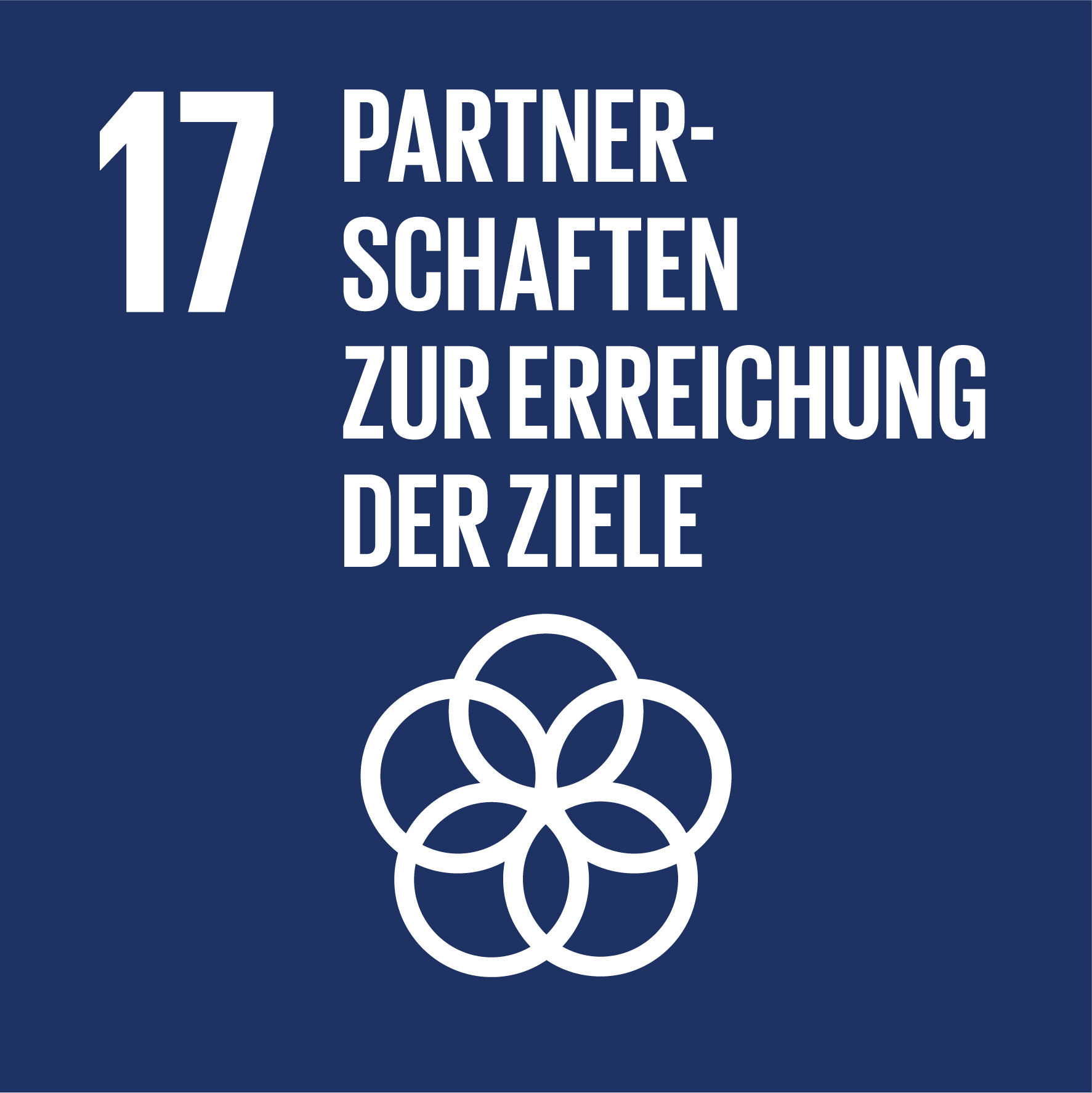
Beteiligte Personen
Projektleitung Hochschule Landshut:
Prof. Dr. Markus Mock
Wissenschaftliche Mitarbeitende:
Raja Sekar Shantha Kumar
FRUTILLA
Frostvorhersage für den Obstanbau durch maschinelles Lernen und Sensorsynthese
Das Ziel von FRUTILLA ist die Entwicklung eines virtuellen Sensornetzwerkes zur Analyse des Mikroklimas auf Ackerflächen. So sollen die Auswirkungen von Wetterbedingungen und Klimawandel auf die Produktion von Biomasse mit hoher räumlicher und zeitlicher Auflösung überwacht werden. Zum Nachweis der effizienten und praktischen Durchführbarkeit untersuchen wir als konkreten Anwendungsfall die Vermeidung von Frostschäden in der Obstproduktion. Dies ist in das Programmziel, Produktionssysteme innerhalb von Ökosystemen zu verstehen und den ökologischen Fußabdruck der Biomasseproduktion zu reduzieren Feinmaschige Datenerfassung und Vorhersage ist aus zwei Hauptgründen von entscheidender Bedeutung. Einerseits müssen Frostschutzmaßnahmen rechtzeitig und nur wenn notwendig durchgeführt werden. Andererseits ist es aus sozialen Gründen auch wichtig, die Erzeuger mit genügend Vorlaufzeit zu benachrichtigen. Dabei sollen sowohl falsch negative Ergebnisse, d. h. kein Warnereignis trotz Frost, als auch falsch positive Ergebnisse, d.h. unnötige Warnungen ausgeschlossen werden.
Insbesondere strebt FRUTILLA an, die Anzahl falsch positiver Warnungen zu reduzieren, da diese zu einem unnötigen Verbrauch natürlicher Ressourcen und CO2-Emissionen durch unnötige Schutzmaßnahmen führen. Dabei darf jedoch die Präzision korrekt positiver Warnungen nicht beeinträchtigt werden.
Die engmaschige Überwachung der Wetterbedingungen erfolgt durch die Synthese von Sensordaten. Dabei werden durch den Aufbau von Modellen für maschinelles Lernen, aus den Daten bestehender Wetterstationen weitere Messwerte synthetisiert, um so feinmaschige Daten für Anbauflächen zu bekommen die keine eigenen Wetterstationen haben.
Am Ende des Projekts wird unser System die bestehenden Wetterstationen mit vielen synthetisierten Sensoren zu einem feinmaschigen Netzwerk für eine zeitnahe und präzise Vorhersage der zu erwartenden Frostbedingungen ergänzt haben.